Note
Click here to download the full example code
SKLearn and SVMΒΆ
We apply dman
to an example on SVM in the SK Learn package.
You can find the sklearn
example this one is based on here.
It considers a non-linear binary classification problem, which is solved using SVC with an RBF kernel.
We will not go into details on the classification problem, but instead
show how one can use dman
to store the generated data.
We show the basic example code with some data-structures added already, which we will use later for storage.
import numpy as np
import matplotlib.pyplot as plt
from sklearn import svm
import dman
@dman.modelclass(storable=True)
class Config:
nb_samples: int = 300
resolution: int = 500
seed: int = 0
@dman.modelclass(storable=True)
class Samples:
X: dman.barray = dman.recordfield(stem='x-samples', subdir='samples')
Y: dman.barray = dman.recordfield(stem='y-samples', subdir='samples')
def generate_samples(cfg: Config):
np.random.seed(cfg.seed)
X = np.random.randn(cfg.nb_samples, 2)
Y = np.logical_xor(X[:, 0] > 0, X[:, 1] > 0)
return Samples(X, Y)
def build_figure(clf: svm.NuSVC, samples: Samples):
fig, ax = plt.subplots(1, 1)
# evaluate the fit
xx, yy = np.meshgrid(np.linspace(-3, 3, 500), np.linspace(-3, 3, 500))
Z = clf.decision_function(np.c_[xx.ravel(), yy.ravel()])
Z = Z.reshape(xx.shape)
# show the result
ax.imshow(
Z,
interpolation="nearest",
extent=(xx.min(), xx.max(), yy.min(), yy.max()),
aspect="auto",
origin="lower",
cmap=plt.cm.PuOr_r,
)
ax.contour(xx, yy, Z, levels=[0], linewidths=2, linestyles="dashed")
ax.scatter(samples.X[:, 0], samples.X[:, 1], s=30, c=samples.Y, cmap=plt.cm.Paired, edgecolors="k")
ax.set_xticks(())
ax.set_yticks(())
ax.axis([-3, 3, -3, 3])
return fig
We can then run the experiment and plot the result as follows
cfg = Config()
samples = generate_samples(cfg)
clf = svm.NuSVC(gamma="auto")
clf.fit(samples.X, samples.Y)
build_figure(clf, samples)
plt.show()
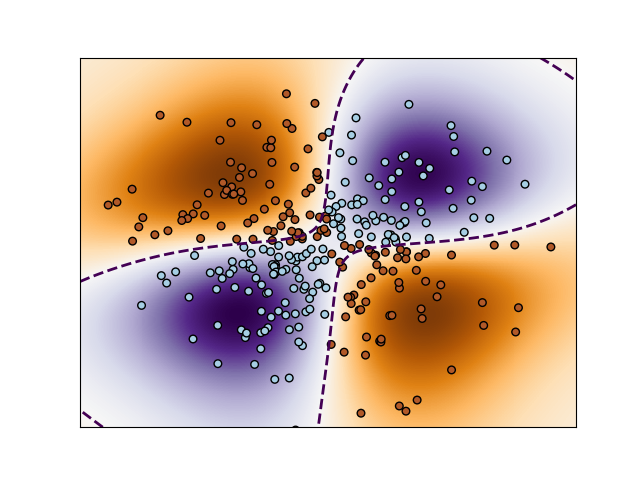
To make the NuSVC
instance serializable we use a template. Luckily the
NuSVC`
class is entirely defined by its __dict__
.
@dman.modelclass(storable=True)
class T_NuSVC:
store: dman.mdict
@classmethod
def __convert__(cls, other: svm.NuSVC):
store = dman.mdict(store_by_key=True, subdir='svm-data')
for k, v in other.__dict__.items():
if isinstance(v, np.ndarray):
v = v.view(dman.numeric.barray) # store as binary files
store[k] = v
return cls(store=store)
def __de_convert__(self):
res = svm.NuSVC()
res.__dict__ = {k: v for k, v in self.store.items()}
return res
dman.serializable(svm.NuSVC, template=T_NuSVC)
dman.storable(svm.NuSVC)
We create a data-type gathering everything together
@dman.modelclass
class Result:
cfg: Config = dman.recordfield(stem='config')
samples: Samples = dman.recordfield(stem='samples', subdir='data')
clf: svm.NuSVC = dman.recordfield(stem='svm', subdir='data')
And can then save the data as follows:
res = Result(cfg, samples, clf)
_ = dman.save('result', res)
The resulting file structure looks like:
dman.tui.walk_directory(dman.mount('result'), show_content=True)
π .dman/cache/examples:cases:example_svm/result
β£ββ π data
β β£ββ π samples
β β β£ββ π x-samples.npy (4.9 kB)
β β βββ π y-samples.npy (428 bytes)
β β£ββ π _dual_coef_.npy (1.4 kB)
β β£ββ π _intercept_.npy (136 bytes)
β β£ββ π _n_support.npy (136 bytes)
β β£ββ π _num_iter.npy (132 bytes)
β β£ββ π _probA.npy (128 bytes)
β β£ββ π _probB.npy (128 bytes)
β β£ββ π class_weight_.npy (144 bytes)
β β£ββ π classes_.npy (130 bytes)
β β£ββ π dual_coef_.npy (1.4 kB)
β β£ββ π intercept_.npy (136 bytes)
β β£ββ π n_iter_.npy (132 bytes)
β β£ββ π samples.json (360 bytes)
β β ββββββββββββββββββββββββββββββββββββββββββββββββββββββββββββββββββββββ
β β {
β β "X": {
β β "_ser__type": "_ser__record",
β β "_ser__content": {
β β "target": "samples/x-samples.npy",
β β "sto_type": "_num__barray"
β β }
β β },
β β "Y": {
β β "_ser__type": "_ser__record",
β β "_ser__content": {
β β "target": "samples/y-samples.npy",
β β "sto_type": "_num__barray"
β β }
β β }
β β }
β β ββββββββββββββββββββββββββββββββββββββββββββββββββββββββββββββββββββββ
β β£ββ π support_.npy (744 bytes)
β β£ββ π support_vectors_.npy (2.6 kB)
β βββ π svm.json (4.4 kB)
β ββββββββββββββββββββββββββββββββββββββββββββββββββββββββββββββββββββββ
β {
β "store": {
β "_ser__type": "_ser__mdict",
β "_ser__content": {
β "store": {
β "decision_function_shape": "ovr",
β "break_ties": false,
β "kernel": "rbf",
β "degree": 3,
β "gamma": "auto",
β "coef0": 0.0,
β "tol": 0.001,
β "C": 0.0,
β "nu": 0.5,
β "epsilon": 0.0,
β "shrinking": true,
β "probability": false,
β "cache_size": 200,
β "class_weight": null,
β "verbose": false,
β "max_iter": -1,
β "random_state": null,
β "_sparse": false,
β "n_features_in_": 2,
β "class_weight_": {
β "_ser__type": "_ser__record",
β "_ser__content": {
β "target": "class_weight_.npy",
β "sto_type": "_num__barray"
β }
β },
β "classes_": {
β "_ser__type": "_ser__record",
β "_ser__content": {
β "target": "classes_.npy",
β "sto_type": "_num__barray"
β }
β },
β "_gamma": 0.5,
β "support_": {
β "_ser__type": "_ser__record",
β "_ser__content": {
β "target": "support_.npy",
β "sto_type": "_num__barray"
β }
β },
β "support_vectors_": {
β "_ser__type": "_ser__record",
β "_ser__content": {
β "target": "support_vectors_.npy",
β "sto_type": "_num__barray"
β }
β },
β "_n_support": {
β "_ser__type": "_ser__record",
β "_ser__content": {
β "target": "_n_support.npy",
β "sto_type": "_num__barray"
β }
β },
β "dual_coef_": {
β "_ser__type": "_ser__record",
β "_ser__content": {
β "target": "dual_coef_.npy",
β "sto_type": "_num__barray"
β }
β },
β "intercept_": {
β "_ser__type": "_ser__record",
β "_ser__content": {
β "target": "intercept_.npy",
β "sto_type": "_num__barray"
β }
β },
β "_probA": {
β "_ser__type": "_ser__record",
β "_ser__content": {
β "target": "_probA.npy",
β "sto_type": "_num__barray"
β }
β },
β "_probB": {
β "_ser__type": "_ser__record",
β "_ser__content": {
β "target": "_probB.npy",
β "sto_type": "_num__barray"
β }
β },
β "fit_status_": 0,
β "_num_iter": {
β "_ser__type": "_ser__record",
β "_ser__content": {
β "target": "_num_iter.npy",
β "sto_type": "_num__barray"
β }
β },
β "shape_fit_": [
β 300,
β 2
β ],
β "_intercept_": {
β "_ser__type": "_ser__record",
β "_ser__content": {
β "target": "_intercept_.npy",
β "sto_type": "_num__barray"
β }
β },
β "_dual_coef_": {
β "_ser__type": "_ser__record",
β "_ser__content": {
β "target": "_dual_coef_.npy",
β "sto_type": "_num__barray"
β }
β },
β "n_iter_": {
β "_ser__type": "_ser__record",
β "_ser__content": {
β "target": "n_iter_.npy",
β "sto_type": "_num__barray"
β }
β }
β },
β "subdir": "svm-data",
β "store_by_key": true
β }
β }
β }
β ββββββββββββββββββββββββββββββββββββββββββββββββββββββββββββββββββββββ
β£ββ π config.json (63 bytes)
β ββββββββββββββββββββββββββββββββββββββββββββββββββββββββββββββββββββββββββ
β {
β "nb_samples": 300,
β "resolution": 500,
β "seed": 0
β }
β ββββββββββββββββββββββββββββββββββββββββββββββββββββββββββββββββββββββββββ
βββ π result.json (650 bytes)
ββββββββββββββββββββββββββββββββββββββββββββββββββββββββββββββββββββββββββ
{
"_ser__type": "Result",
"_ser__content": {
"cfg": {
"_ser__type": "_ser__record",
"_ser__content": {
"target": "config.json",
"sto_type": "Config"
}
},
"samples": {
"_ser__type": "_ser__record",
"_ser__content": {
"target": "data/samples.json",
"sto_type": "Samples"
}
},
"clf": {
"_ser__type": "_ser__record",
"_ser__content": {
"target": "data/svm.json",
"sto_type": "NuSVC"
}
}
}
}
ββββββββββββββββββββββββββββββββββββββββββββββββββββββββββββββββββββββββββ
We can load the experiment and show the result once more
res = dman.load('result')
build_figure(res.clf, res.samples)
plt.show()
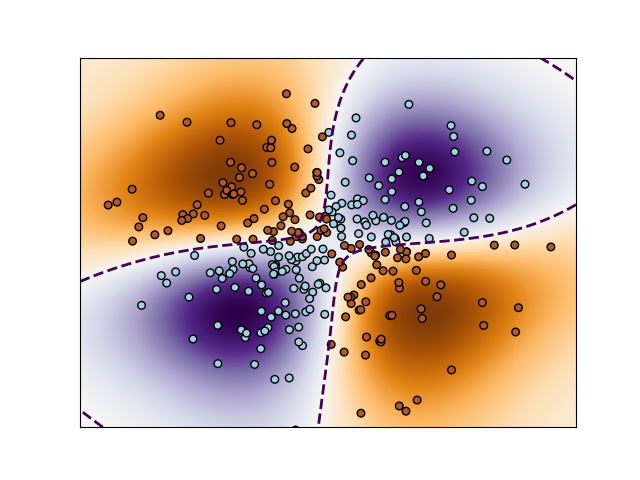
Total running time of the script: ( 0 minutes 5.978 seconds)